In this line of work we develop visual interfaces that help users identify and correct biases in algorithmic decision making systems. We typically use causal models and do so in two ways: (1) as explainable models that encode black box models, such as neural networks, and give insights into how certain decisions come about, and (2) as interpretable models that can operate as fully functional and tunable algorithmic decision systems. Since bias and fairness are quite subjective and mean different things to different people, the systems developed in this research typically have well developed interactive visual interfaces that put the human in the loop to take on an active role in the bias mitigation process.
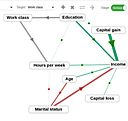 |
D-BIAS: A Causality-Based Human-in-the-Loop System for Tackling Algorithmic Bias
B. Ghai, K. Mueller
IEEE Trans. on Visualization and Computer Graphics (Special Issue IEEE VIS 2022)
29(1):473-482, 2023
|
 |
 |
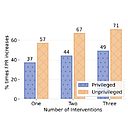 |
Cascaded Debiasing: Studying the Cumulative Effect of Multiple Fairness-Enhancing Interventions
B. Ghai, M. Mishra, K. Mueller
ACM Conference on Information and Knowledge Management (CIKM)
Atlanta, GA, October, 2022 |
 |
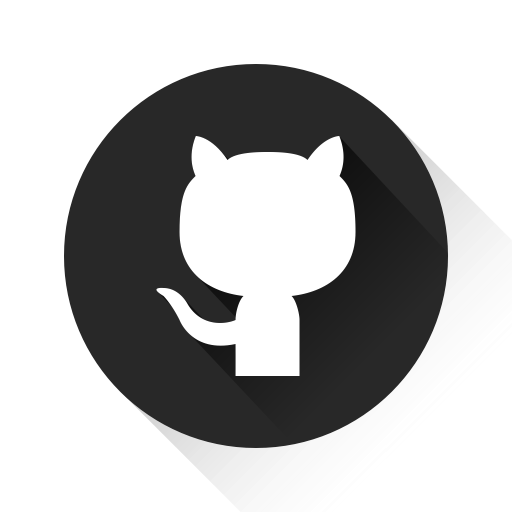 |
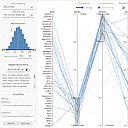 |
WordBias: An Interactive Visual Tool for Discovering Intersectional Biases Encoded in Word Embeddings
B. Ghai, Md. N. Hoque, K. Mueller
ACM CHI Late Breaking Work
Virtual, May 8-13, 2021 |
 |
 |
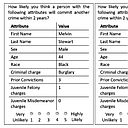 |
Measuring Social Biases of Crowd Workers using Counterfactual Queries
B Ghai, QV Liao, Y Zhang, K Mueller
Fair & Responsible AI Workshop (co-located with CHI 2020)
Virtual, April 2020 |
 |
|
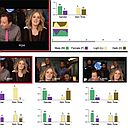 |
Toward Interactively Balancing the Screen Time of Actors Based on Observable Phenotypic Traits in Live Telecast
Md. N Hoque, S. Billah, N. Saquib, K. Mueller
ACM Conference on Computer-Supported Cooperative Work and Social Computing (CSCW)
Virtual, October 17-21, 2020 |
 |
 |